NSF announces $40 million for AI-powered protein design applications
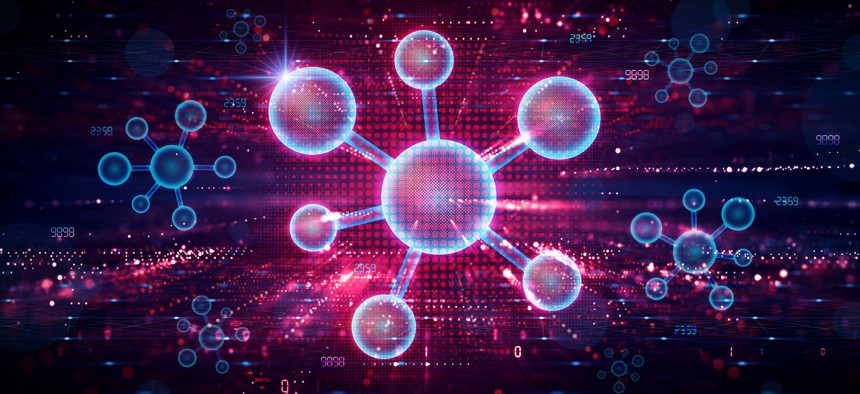
ArtemisDiana/Getty Images
The National Science Foundation wants researchers across disciplines to develop applications for artificial intelligence that boost researchers’ advancements in protein design.
The National Science Foundation is looking to foster more research and innovation in applications that artificial intelligence may have in protein design — a field of biotechnology that offers potential advances across several fields, like medicine and environmental protection.
The NSF committed $40 million in new funding in late February to facilitate new approaches in protein design that leverage AI technologies under the agency’s Use-Inspired Acceleration of Protein Design — or USPRD — initiative.
On its own, protein design aims to create novel proteins and engineer them to perform certain biological functions. Such efforts have major implications for medical therapies, like vaccine creation and disease treatment, as well as for environmental solutions, like breaking down plastics with enzymes.
This new series of funding will be dispersed over the course of three years within the USPRD, focusing on enzyme design and how AI can further enable this research.
“What we're hoping this program is going to do is, to some degree, democratize these capabilities, so that other people that have a need for proteins and specific applications are able to basically use these tools,” David Tennenhouse, a senior advisor in the NSF’s Technology, Innovation and Partnerships Directorate, told Nextgov/FCW. “One of the two areas we're really focusing on is enzymes, because enzymes are a type of protein that are really key to any number of types of chemical reactions that can enable a lot of different applications.”
Successful protein design hinges on being able to understand the structure of a given protein. Advanced computing power has accelerated researchers’ ability to view and understand protein characteristics. Tennenhouse explained that researchers are able to house protein sequences in databases, and that AI capabilities can facilitate the design process even further.
“There's this very large protein database, and that means that we have huge amounts of training data that tells us things about these proteins,” Tennenhouse said. “We also now have, with machine learning software…people are very much able to say, for a given sequence, here's what the shape is going to be.”
He said that the use of AI and machine learning models can provide accurate predictions about the structure of protein, given its DNA sequence.
“There's multiple types of software [that] together are able to give researchers a really good handle on that,” he said. “The next step is really to be able to get a handle on ‘okay, now, how does that affect the function?’ Because what you really want to do is start now designing and engineering proteins to do the things that you want to do.”
Tennenhouse added that the software used in protein design is more on the machine learning component of AI — relying more on pattern-recognition capabilities than generative output.
“This is very application-specific machine learning pattern recognition,” he said.
In keeping with the Biden administration’s larger human-centric approach to AI use and deployment, the NSF is looking for a diverse cohort of perspectives to participate in its USPRD initiative and fuel protein design application in a variety of fields.
"NSF aims to build on these research advances by announcing the USPRD opportunity for experts from academia and industry to jointly address the significant barriers to extending this work to enzyme design and translating it into widespread use," Thyaga Nandagopal, division director for the TIP Division of Innovation and Technology Ecosystems, said in the press release.
Tennenhouse added that the human scientific component is as crucial as the machine learning advantage that TIP and USPRD are looking for.
“AI algorithms, they're not doing the whole show today,” he said. “You have human protein designers. They are using these algorithms to get candidates…so it's really the AI letting you kind of zero-in on the better opportunities, and you still have researchers who are engaging with the data with other information that they have and with results that they get in labs.”